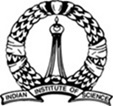 |
Modelling urban dynamics in rapidly urbanising Indian cities
a Energy & Wetlands Research Group, Center for Ecological Sciences [CES], Indian Institute of Science, Bangalore, Karnataka 560 012, India b Centre for Sustainable Technologies (astra), Indian Institute of Science, Bangalore, Karnataka 560 012, India c Centre for infrastructure, Sustainable Transportation and Urban Planning [CiSTUP], Indian Institute of Science, Bangalore, Karnataka 560 012, India d RCGSIDM, IIT Kharagpur, Kharagpur 721302, India
| 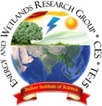 |
|
Data and method
Temporal remote sensing data of Landsat TM and ETM + downloaded from GLCF (http://glcf.umd.edu/data http://www.usgs.gov) were preprocessed to correct geometrical and radiometric integrity. Remote sensing data were supplemented with the Survey of India topographic maps (of 1:50,000 and 1:250,000 scale), which were used to generate base layers of the administrative boundary, drainage network, road network etc. Slope map was extracted using ASTER data (30 m) downloaded from USGS (www.usgs.govGround control points (GCPs) and training data were collected using pre calibrated Global Positioning System (GPS) and virtual This is an open access article under the CC BY-NC-ND license (http://creativecommons.org/licenses/by-ncnd/4.0/). online spatial maps such as Bhuvan (http://bhuvan.nrsc.gov.in) and Google Earth (http://earth.google.com). GCPs were useful in geometric rectification of remote sensing data. Census data (1991, 2001 and 2011) was used to understand population dynamics.
2.1. Modelling of urbanisation and sprawl (procedure described in Fig. 2) involved:
Data generation, Land use and land cover analysis and integrated model generation and validation.

Fig. 2. Method for spatial data analysis, metrics and modelling.
2.2.Data creation:
Data were obtained from survey of India topographic sheets. The sheets with scale 1:50,000 and 1:2,50,000 were scanned with high resolution. City administrative maps were obtained from respective metropolitan development authority database. Ground truth data of various locations within the study region were considered using hand held GPS. Places which were inaccessible and remote were surveyed with recent data of Google earth and Bhuvan. All these data sets corresponding to different study regions were geo-referenced and re-projected to common datum world geodetic system 1984 (WGS84) and respective Universal Transverse Mercator (UTM) zones to ensure uniformity in mapping. Hence region specific administrative boundary maps were obtained. Further, 10 km buffer were drawn from centroid of central business district (CBD) to better understand the urbanisation process beyond city administrative boundary.
2.3. Land use analysis:
Satellite data for various time periods were geo-referenced (to WGS84 and respective UTM zones), geocorrected, rectified and cropped pertaining to different study regions. To maintain similar resolution and for better comparison, satellite data were resampled to 30 m using nearest neighbourhood function. Land use analysis was performed to understand change in landscape pattern throughout the study regions temporally. It involved the following process – a) Generation of false color composite (FCC) image b) Digitizing training polygons using FCC as base layer to distinguish heterogeneous features c) Collection of training polygons using Google earth (https://www.google.com/earth) used as ancillary data for classification, d) Classification using Gaussian Maximum Likelihood (GML) classifier, based on probability and cost functions (Duda et al., 2000; Ramachandra et al., 2012) Land use with gradient analysis done earlier (Ramachandra et al., 2014a; Ramachandra et al., 2014b,c,d; Chandan et al., 2014) aided in understanding the behaviour of agents at local levels, which were used to model and predict urban growth. Historical land use details with transition probabilities were used to model and visualise urban growth in five major cities. Land use analysis was performed using geographical analysis support system (GRASS) open source software as four different categories as shown in Table 1.
Category |
Features involved |
Built up |
Houses, buildings, road features, paved surfaces etc. |
Vegetation |
Trees, Gardens and forest. |
Water body |
Sea, Lakes, tanks, river and estuaries |
Others |
Fallow/barren land, open fields, quarry site, dry river/lake basin etc. |
Table 1: List of different categories used for land use analysis and corresponding features.
Classification process is complete only when its accuracy is tested. Accuracy can be obtained by preparation of error matrix or confusion matrix. User accuracy, producer accuracy, overall accuracy and kappa statistics were calculated from error matrix. Overall accuracy considers only diagonal elements of reference map and classified map whereas kappa statistic takes offdiagonal elements also into account (Lillesand and Kiefer, 2005).
Integrated model generation and validation: Urbanizing agents and constraints were delineated in the form of points, lines and polygons using Google earth interface. Proximity maps were generated
using minimum and maximum distance functions from each agent layers. Data values were normalized using fuzzy functions and the entire range were between 0 and 255, 0 indicating no changes and 255 indicating maximum probability of change in land use types. Analytical hierarchical process (AHP) was employed to estimate Principal Eigen vector and therefore priority maps were created. Classified land use maps for initial stages say, T0,T1and T2were considered along with slope and drainage layers analysed using DEM imageries were given as inputs to generate land use transitions from T0 to T1 and T1to T2. Markov chain analysis was used to calculate transition probability matrix based on two different time periods of classified images, where probability of each land use type changing to remaining other categories within the region considered is recorded after specific number of iterations. Cellular automation with Markov chain was used to predict future land use for a specified year. Model validation was done by generating kappa statistics for time period T2 of both classified image versus simulated image.
Modelling using FUZZY AHP-CA: Integration of Fuzzy Logic, Analytical Hierarchical Process (AHP), Multi Criteria Evaluation (MCE), Markov chains and Cellular Automata (CA) were used to model and visualise the urban growth. Agents (roads, industries educational institutions, bus stands, railway stations, metro, population, etc.) of urbanisation were normalized. Water bodies considered as constraints as they are identified as conservation regions (in CDP: city development plan). The fuzzy based analysis is used to normalize the contributing factors between 0 and 255, where 255 showing the maximum probability of change and 0 indicating no change, for different land uses. The model is a multiple input and based on fuzzy rules. Each agent that has been added for creating fuzziness represents each rule that contribute for determining the factors of urbanisation. With a N-Dimensional space, withn dimensions an image set A is then described using a Gaussian membership function as per Eq. (1)
μd(Xn) = exp ((Xn-vn)2/2Sn2) (1)
where vd is a pixel location for the nthagent and s is this agent’s deviation.
Further these create fuzzy sets rules. For example, if E is a fuzzy set, an output of all inputs of S rules, fuzzy set μe where s belongs to S with different decision of agents X and denoted as in Eq. (2)
μE(X) = ⊕1SμE (s) (2)
Fuzzy sets are defuzzified to obtain values that represent the influence of agents. Fuzzy results provide us details of influence of each agents through the nodal values that are defuzzified based on rating that are based on perspective of modelling criteria based on gradients of influence. This is in the numerical form of influence is considered as an input to AHP. This essential indicates people’s perception of thinking and ways that they can interact in urban growth. The values of agents were considered as input to AHP to determine the weights of driving factors using pair wise comparisons ith weights as Eigen vectors. The weights analysed and calibrated through AHP is verified using measured consistency ratio (CR). CR below 0.1, the model is consistent and used for subsequent processes. These weights along with the factors of growth are combined along with use of constraints to obtain site suitability maps for different land uses using Eq. (3).
----------------(3)
where LC is the linear combination of weights, n is the number of factors, D decision factor, W is the weight of the factor. Markov chain are used to determine the change probability between two historical datasets to derive the growth in the future scenarios based on different criteria’s. The Markovian transition matrix indicates the probability of the particular land use being converted to other land uses on single time step. Cellular Automata based on the site suitability and the transition matrix is used to spatially predict the changes in land use based on current land use at every single time step, based on the neighbouring pixels. Fuzzy characterises the agents based on human behaviour and thinking with certain rules and analysis, AHP considers the numerical form of fuzzy behaviour to obtain the most and least influential agents for every point in the study region. These modules act to convey the agent based influence on the land use. CA-Markov is used to understand the spatial allocation of each cell based on rules and neighbourhood as to how each change and adapt in changing environs. This integration of agents to conventional modelling using CA Markov is understood using case studies of five cities of India. Validation of the simulated datasets were performed with classified datasets through kappa indices, as a measure of agreement. Once these data and agents are trained and validated, data is used to model and simulate for future trends (ten years) with definite time steps.
|
Citation : H.A. Bharath, M.C. Chandan, S. Vinay, T.V. Ramachandra, 2018. Modelling urban dynamics in rapidly urbanising Indian cities. The Egyptian Journal of Remote Sensing and Space Science, Volume 19, Issue 2, December 2016, Pages 175-193, https://doi.org/10.1016/j.ejrs.2016.09.001
* Corresponding author
H.A. Bharath
Ranbir and Chitra Gupta School of Infrastructure
Design and Management (RCGSIDM), Twin Science Block, IIT-Kharagpur, Kharagpur,
West Bengal 721302, India.
E-mail : bharathhaithal@gmail.com
|
|
|